Calculate Kl Divergence Continuous From Data
Given two distributions $p(x)$ and $q(x)$, the Kullback-Leibler divergence is defined as
$$ D_\text{KL}(p(x) \parallel q(x) ) = \int_{-\infty}^\infty p(x) \log\left(\frac{p(x)}{q(x)}\right)\, dx = \mathbb E_{p(x)} \left[\log\left(\frac{p(x)}{q(x)}\right) \right]. $$
Kullback-Leibler divergence is also called relative entropy. In fact, KL divergence can be decomposed into cross entropy $H(p) = -\int_{-\infty}^\infty p(x) \log q(x), dx$ and entropy $H(p) = -\int_{-\infty}^\infty p(x) \log p(x), dx$
$$ D_\text{KL}(p \parallel q) = \int_{-\infty}^\infty p(x) \log p(x)\, dx - \int_{-\infty}^\infty p(x) \log q(x) \, dx = - H(p) + H(p, q), $$
which is
$$ D_\text{KL}(p \parallel q) + H(p) = H(p, q). $$
What does it mean?
We assume two uniform distributions,
$$ p(x) = \begin{cases} 1/\Delta_p & \text{for } x\in [0, \Delta_p] \\ 0 & \text{else} \end{cases} $$
and
$$ p(x) = \begin{cases} 1/\Delta_q & \text{for } x\in [0, \Delta_q] \\ 0 & \text{else}. \end{cases} $$
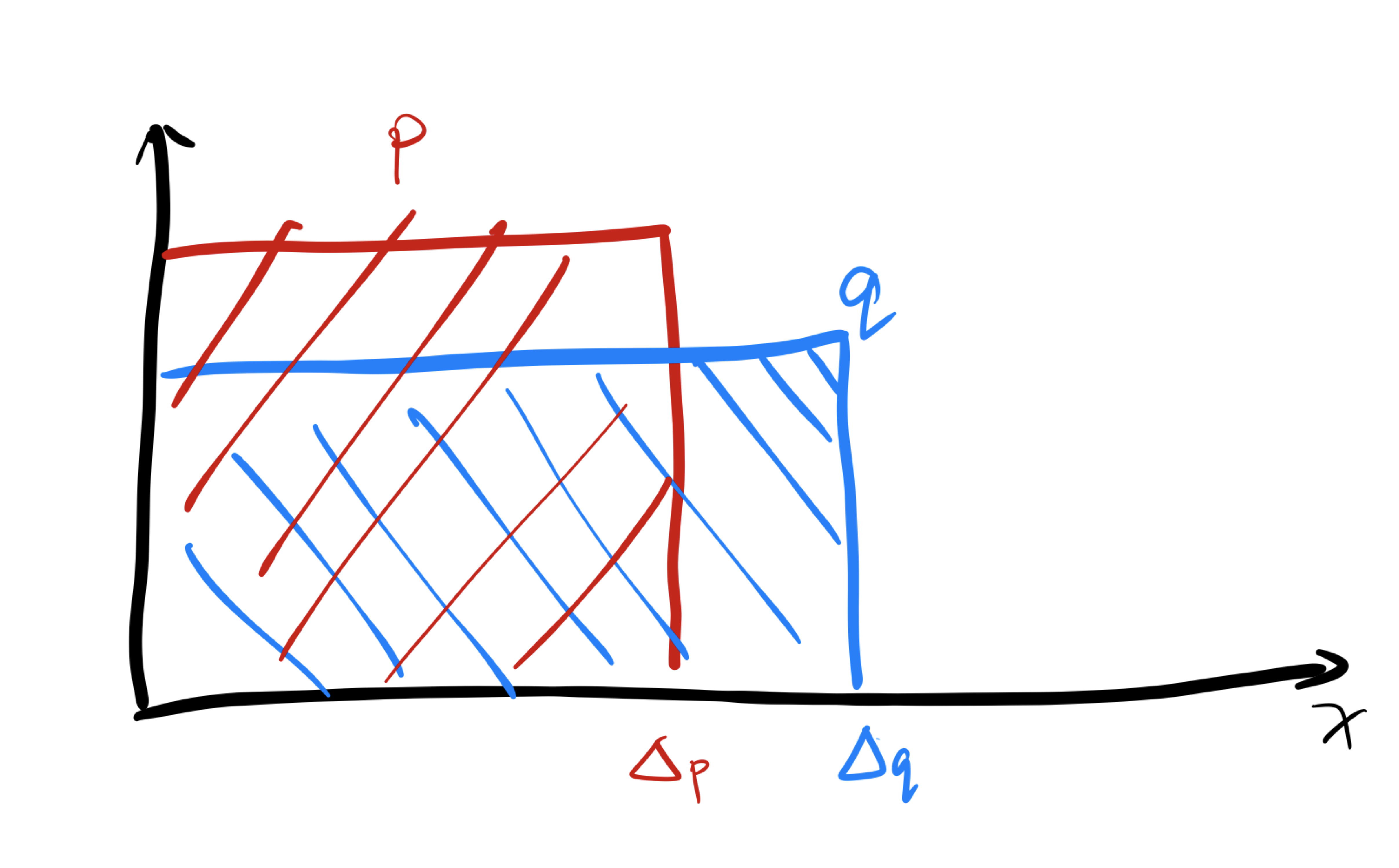
We will assume $\Delta_q > \Delta_p$ for simplicity. The KL divergence is
$$ D_\text{KL}(p\parallel q) = \int_0^{\Delta_p} \frac{1}{\Delta_p}\log\left( \frac{1/\Delta_p}{1/\Delta_q} \right) dx +\int_{\Delta_p}^{\Delta_q} \lim_{\epsilon\to 0} \epsilon \log\left( \frac{\epsilon}{1/\Delta_q} \right) dx, $$
where the second term is 0. We have the KL divergence
$$ D_\text{KL}(p\parallel q) = \log\left( \frac{\Delta_q}{\Delta_p} \right). $$
The KL divergence is 0 if $\Delta_p = \Delta_q$, i.e., if the two distributions are the same.
KL Divergence of Two Gaussians
Assuming the two distributions are two Gaussians $p(x)=\frac{1}{\sigma_p\sqrt{2\pi}} \exp\left( - \frac{ (x - \mu_p)^2 }{2\sigma_p^2} \right)$ and $q(x)=\frac{1}{\sigma_q\sqrt{2\pi}} \exp\left( - \frac{ (x - \mu_q)^2 }{2\sigma_q^2} \right)$, the KL divergence is
$$ D_{\text{KL}}(p\parallel q) = \mathbb{E}_p \left[ \log \left(\frac{p}{q}\right) \right]. $$
Notice that
$$ \log \left( \frac{p}{q} \right) = \log \left( \exp\left( - \frac{ (x - \mu_p)^2 }{2\sigma_p^2} + \frac{ (x - \mu_q)^2 }{2\sigma_q^2} \right) \right). $$
As a simpler version of the problem, we assume that $\sigma_p = \sigma_q = \sigma$, so that
$$ \log \left( \frac{p}{q} \right) = \frac{ -(x - \mu_p)^2 + (x - \mu_q)^2 }{2\sigma^2} = \frac{ \mu_q^2 - \mu_p^2}{2\sigma^2} + \frac{ (\mu_p - \mu_q) x }{\sigma^2}. $$
The KL divergence becomes
$$ D_{\text{KL}} = \mathbb E_p \left[ \frac{ \mu_q^2 - \mu_p^2}{2\sigma^2} + \frac{ (\mu_p - \mu_q) x }{\sigma^2} \right] = \frac{ \mu_q^2 - \mu_p^2}{2\sigma^2} + \frac{ (\mu_p - \mu_q) }{\sigma^2} \mathbb E_p \left[ x \right] = \frac{ (\mu_q - \mu_p)^2}{2\sigma^2}. $$
One could easily see that $\mathbb E_p \left[ x \right]=\mu$ using symmetries. The KL divergence for two Gaussians is symmetric for the distributions.
As an example, we calculate the KL divergence for the example shown in the figure.
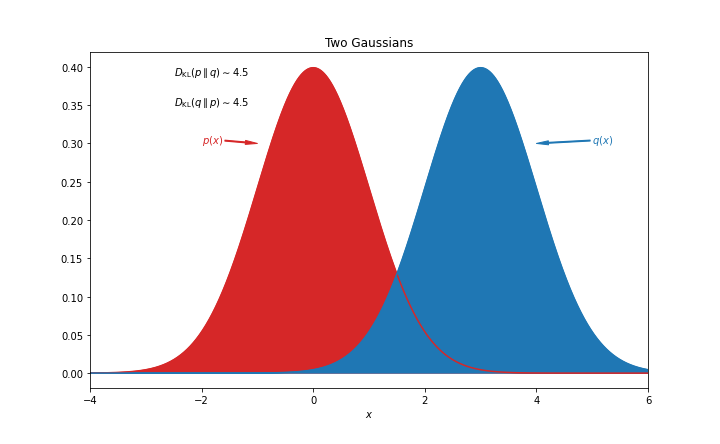
To get some intutions, we calculate the integrant $p(x)\log\left(p(x)/q(x)\right)$. The area under curve will be the KL divergence.
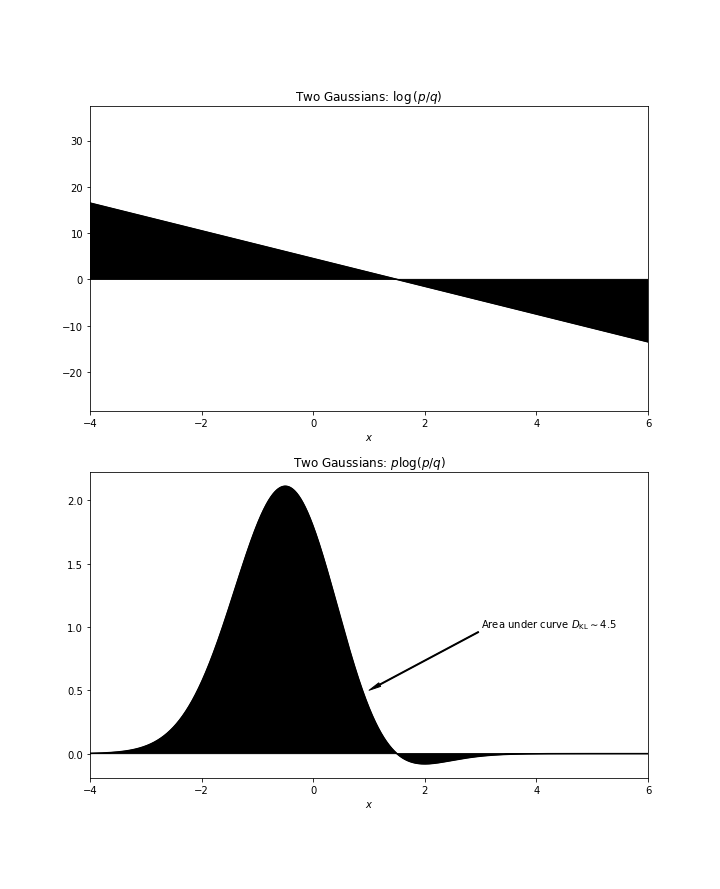
KL Divergence is not necessarily symmetric
Using the uniform distribution example, we realize that
$$ D_\text{KL}(q\parallel p) \to \infty, $$
which is very different from $D_\text{KL}(p\parallel q)$.
To illustrate the asymmetries using continuous , we will calculate the KL divergence of a Gaussian $p(x) = \frac{1}{\sigma\sqrt{2\pi}} \exp\left( - \frac{ (x - \mu_{p})^2 }{2\sigma^2} \right)$ and a Guassian mixture $q(x)=\frac{1}{2\sigma\sqrt{2\pi}} \exp\left( - \frac{ (x - \mu_{q1})^2 }{2\sigma^2} \right) + \frac{1}{2\sigma\sqrt{2\pi}} \exp\left( - \frac{ (x - \mu_{q2})^2 }{2\sigma^2} \right)$. The KL divergence $D_{\text{KL}}(p \parallel q)$ is
$$ D_{\text{KL}}(p \parallel q) = \mathbb{E}_p \left[ \log\left( \frac{p}{q} \right) \right]. $$
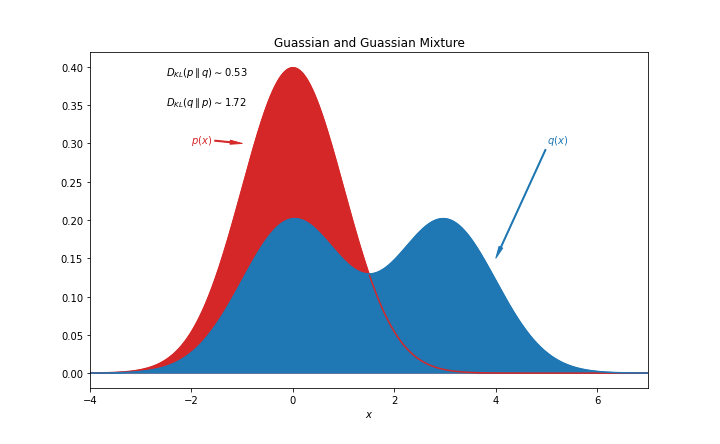
We will calculate the integrants and area under curve will be the KL divergence $D_\text{KL}(p\parallel q)$.
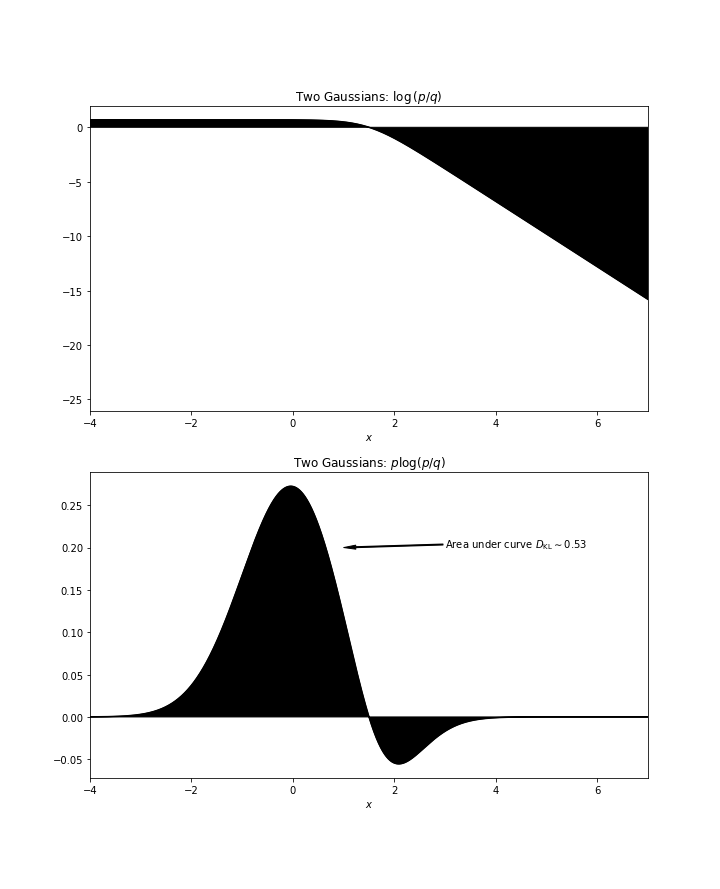
The reverse KL divergence $D_\text{KL}(q\parallel p)$ is different.
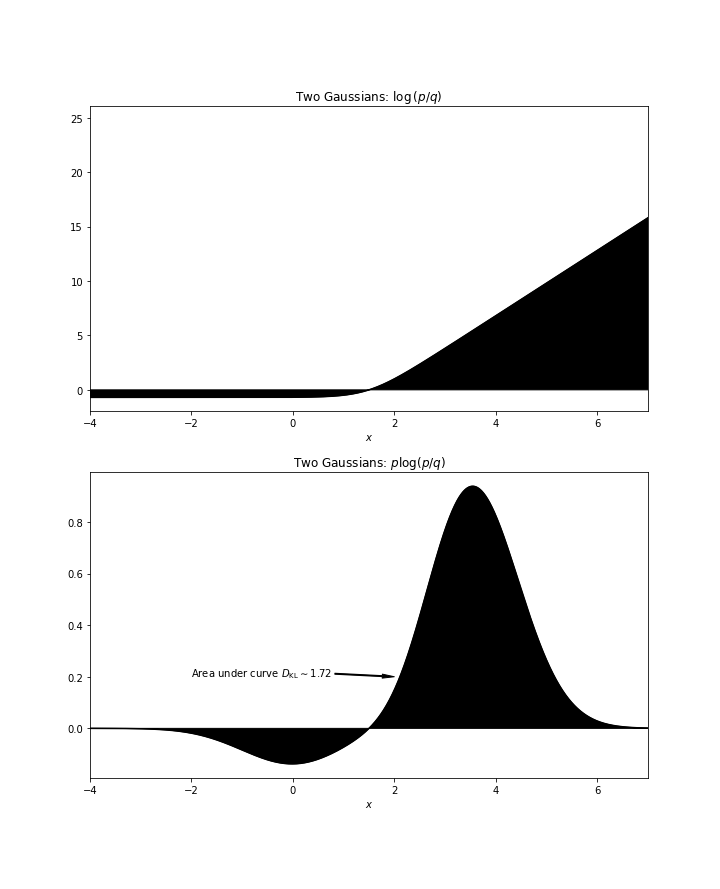
The fact that KL divergence is not symmetric indicates that it can not be a distance measure.
Source: https://datumorphism.leima.is/wiki/machine-learning/basics/kl-divergence/
0 Response to "Calculate Kl Divergence Continuous From Data"
Publicar un comentario